What’s driving legacy modernization?
Over the last five years, organizations have embedded technology to drive every aspect of their business operations. Today, an enterprise runs on hundreds of technology systems, all of which are nearing obsolescence at differing rates.
Legacy modernization becomes a strategic necessity when these systems begin to hinder (rather than enable) teams to accomplish their objectives. At an organizational level, legacy modernization enables enterprises to realize the following advantages:
- Modern systems can scale more easily, and their modularity brings greater agility to the enterprise technology stack.
- Legacy modernization lowers the technical debt while reducing the operating costs associated with business technology.
- It opens the doors for adopting and integrating new digital technologies like Artificial Intelligence (AI), which can enable new revenue streams.
- Aligning the application infrastructure with the latest industry standards empowers enhanced business processes and improved employee experiences.
- Finally, modern technology systems are built to be more secure by design, which lowers exposure to cyber risk.
2023 figures indicate that the top reasons driving legacy modernization include improved security (58%), improved efficiency (54%), cost reductions (49%), ability to integrate with modern technologies (46%), and increased scalability and flexibility (41%).1
What makes legacy modernization challenging?
Despite the known upsides of legacy modernization, nearly 66% of enterprises still use legacy apps to power core operations, and 60% use them for customer-facing processes.2 So, why this disparity?
The answer lies in the challenging nature of legacy modernization. Here are some factors that keep organizations from realizing the advantages of modernizing their legacy systems:
- Lack of comprehension of the legacy tech stack: Most legacy systems that power modern enterprises were developed by teams that no longer work at the organization. The knowledge of these systems is usually siloed and resides with some individuals. In addition, the absence of documentation makes it difficult to understand the underlying architecture and dependencies of these monolithic systems.
- Inability to front large investments of time and money: Legacy modernization is not only an expensive initiative, it can also take months and years to complete. Lack of support from the leadership, and apprehension surrounding the degree of change that such initiatives cause, continue to delay such investments until they become urgent.
- Technological challenges of legacy modernization: Most organizations not only lack the skills required to modernize legacy software, but they are also unable to tap technologies that can accelerate the process and make it less expensive. This includes the use of automation, AI, and accelerators.
However, these challenges can now be mitigated by partnering with technology leaders who exploit Generative AI for easing and accelerating legacy modernization.
A roadmap for accelerating legacy modernization with Generative AI
Generative AI offers some key advantages in some of the most time-consuming and laborious processes that constitute any modernization initiative.
Mapping Generative AI use cases across the legacy modernization pathway
Currently, Generative AI finds applications across all the key phases underpinning a legacy modernization initiative. We mapped these use cases across the four phases of modernization – namely, Analysis, Architecture and Design, Implementation, and Operations and Optimization.
While most applications in the Implementation phase are efficiency-boosting levers, other use cases in the Analysis and Architecture phases can bring strategic advantages like risk mitigation and better prioritization. Generative AI adds value to the four phases of a legacy modernization journey in the following ways –
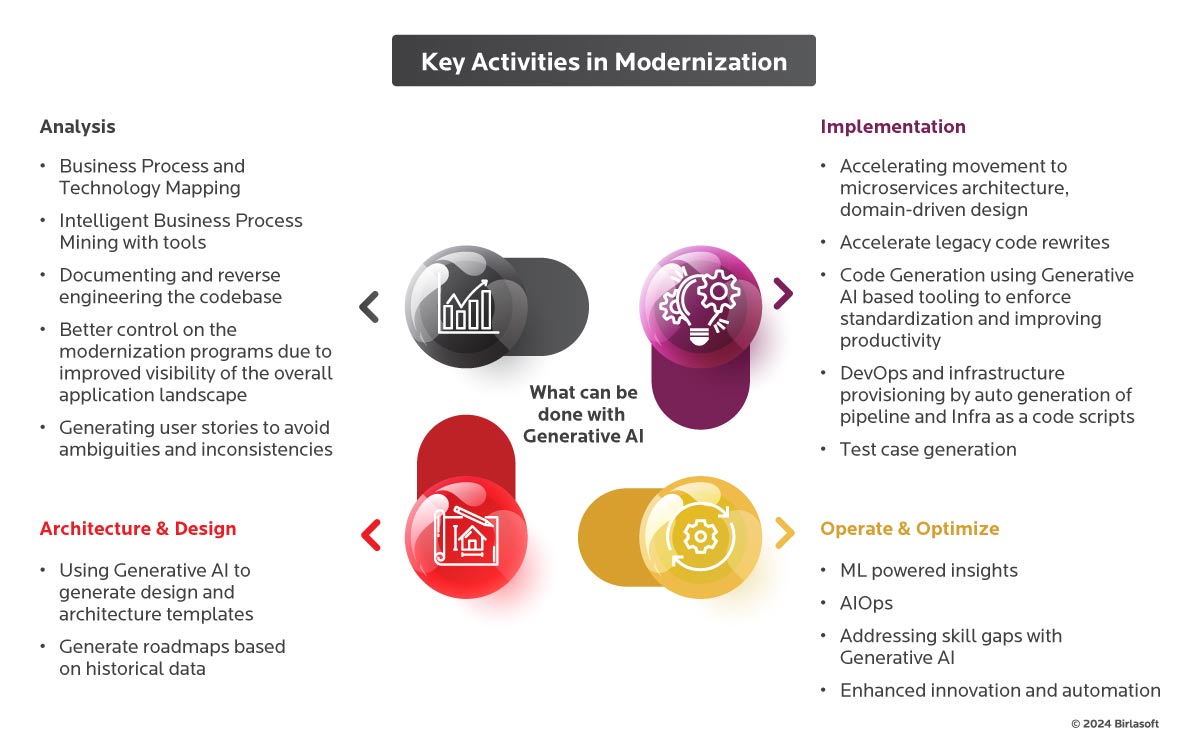
1. Analysis phase
Enterprise technology systems empower business processes in complex and diverse ways. Different teams may use various customizations, and some point solutions may exist as dark IT, thus injecting adoption risks in the later phases.
In this phase, Generative AI can be leveraged to uncover all the applications that support business functions, and to map how business processes get executed within the existing tooling. This can be done by using Generative Adversarial Networks for business process mining, and ultimately, it results in improved visibility over the application landscape driving business operations. These insights inform the modernization objectives, and Generative AI can be used to build rich and complete user stories to set these goals into motion.
2. Architecture and design phase
Defining the target architecture of a system and prioritizing various tasks across the implementation journey strongly impacts the RoI and outcomes of a modernization project. In this context, Generative AI can be leveraged to gain insights into historical data from past modernization projects. These insights can be used to gauge the best possible pathway to attain the desired target state, and how various action items should be prioritized.
Based on these insights, software architects can use Generative AI to rapidly create and iterate upon software architecture templates and design various components of the solution. This accelerates tactical decisions and enables organizations to move swiftly towards their modernization goals.
3. Implementation phase
Once the modernization roadmap has been defined and target architecture finalized, the implementation phase can span multiple months. This introduces the risk of disrupting day-to-day operations, and the implementation consumes considerable man-hours, thus mounting the overall costs of the project.
This is where Generative AI can be exploited to improve efficiency across time-consuming tasks like legacy code rewrites, spinning infrastructure definitions and configurations, and generating test cases. Using Generative AI for code generation enables standardization and improved developer productivity at scale. Ultimately these interventions, combined with insights from the Analysis phase result in accelerated transition to domain-driven design.
4. Operate and optimize phase
While a modernization project may be deemed complete once the implementation is validated, leading organizations view operation of the modernized system as yet another phase of the modernization project. This is because running with the new systems unveils new optimization opportunities, which, when exploited, drive up the RoI of the modernization initiative.
Post-implementation, Generative AI presents some tactical opportunities. This includes the ability to bridge skill gaps by building co-pilot systems that augment the skills of existing employees with validated Generative AI recommendations and insights. Moreover, Generative AI can help IT engineers dig through the metrics and alerts from AIOps platforms to identify optimization opportunities, and to automate the resolution of recurring issues.