Most users feel like they are not getting as much good information from Big Data as they could, or should. To change that, get intimate with your Big Data by thinking about it as a conversation.
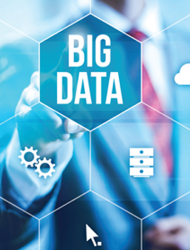
Big Data is an "embarrassment of riches" for most executives, functional managers, & analysts. There is so much great data now available but there is a justifiable feeling of not being able to harvest the value that must be in there. Analytics technology has progressed impressively, but great technology alone won't deliver benefits unless it is applied in a way that brings in new business insights.
The best way to make that connection & solve this Big Data challenge is to use the technology to have a "conversation" with Big Data. To converse with Big Data, there has to be information exchanged in both directions from the user to the data (through the data tools) as well as from the data to the user. Only then can the information extracted from Big Data yield useful insights. This article will explain how to approach a conversation with Big Data, but first let us ensure all the parties to the conversation are speaking the same language.
Big Data and Its Tool Set
Big Data is the huge mass of data that is now available from connected devices and sensors, social media, websites and web applications, and both the industrial and worldwide Internet of Things (IIoT and IoT). Big Data is characterized by its volume, velocity, and variety. About 80 percent of Big Data is unstructured all of which make it a challenge for traditional data management systems and analytical tools.
As might be expected, the focus of technology development at first was simply handling the data. Fortunately, the cloud emerged at the right time to help out. The virtually unlimited expanse of storage available and the highly scalable nature of cloud computing allows companies to gather Big Data so it can be explored, and discard what is not of value without the need for vast local resources large enough to handle peak needs.
Secondarily, technology efforts have shifted to analysis. Users can quickly become overwhelmed by Big Data. There is just so much of it that it exceeds the capacity of our brains and our familiar analysis tools to make sense of it and mine useful intelligence from it. Enterprises already use Business Intelligence applications for internal data inventory, sales, schedules, quality, accounting, finance. Big Data brings in a flood of additional data from other sources, such as adding consumer sentiment data to provide new views and context that could lead to different decisions and actions.
The Big Data challenge is in identifying the right data and turning it into actionable intelligence that can be used to solve a particular problem or gain a competitive advantage. This brings us to the idea of starting a conversation with Big Data, where we figure out the right questions to ask, or even how to get insight from unasked questions.
The Conversation and Competitive Advantage
To absorb and be able to get the most out of Big Data, manufacturing organizations can engage in a "conversational" approach that starts with the use case. The use case describes what we really want to know from the data. For example, a distribution company wanted to know if and how weather affected sales of certain products. Combining detailed sales data (internal information) with weather records (from an external source) the company was able to determine that there was a direct correlation. They were then able to share the information with their manufacturers whose products were affected and with retailers where the products are sold, thus enabling this insight to be factored into forecasts.
Establishing use cases can be challenging because the possibilities are vast. There is debate between managers on what is most important to learn, and because the array of available external data is not well understood, it ends up constraining ideas. To push through the challenge, it can be useful to tie go-to-market strategy and overall business tactics to high-level corporate goals associated with customer value. The unknown variable, which is the availability of specific external data, should be removed from the equation at this stage. It can be factored back in later to evaluate the feasibility of use cases and their prioritization.
Here is an example of a use case involving product design: A manufacturer of gaming technology wanted to know how customers were actually using its gaming machines. The insights would then be embedded into next-generation designs. For this conversation, they combined enterprise data with machine data from the current generation of machines in the field (in casinos) and queried the data about usage patterns, volume, duration of play, and other factors to learn which characteristics and features were most appealing to players. This Big Data conversation formed the basis of a sustained innovation program.
What we don't know comes into play for this next use case: the "unasked question." The conversation in this case starts by focusing on areas of interest rather than on a specific set of data. This conversation might last longer than the highly focused search for a specific answer because it will likely be extended through several iterations. Start by concentrating on types of data that monitor the general area of interest. Let the tools find patterns and clusters of information that grab your attention or spark your curiosity. Refine the use case to explore that interest area more specifically or from another angle. And continue in this manner until actionable intelligence emerges. This is analogous to a cocktail party conversation where strangers will discuss general topics until something of common interest emerges, after which the conversation narrows and deepens.
The conversation obviously should not end there. It might be beneficial to return to general topics again and again to seek out new commonalities and new opportunities. Or the refinement process could offer clues to other common interests that can become important as new patterns are discovered.
The key point here is that wide variations in the types of conversations companies can have with Big Data drive competitive advantage. If your cases are more relevant, creative, or forward-thinking than the competition, you can gain an edge. If your relationships in the ecosystem provide you with more sources of data, or you recognize a possible correlation with external data faster than the other guy, then this might be just the competitive advantage you need.
A Broad Array of Analytic Tools
The sheer volume and variety of Big Data make it difficult if not impossible for the human mind to grasp the hidden information and intelligence buried within. Analytics can convert massive quantities of data into highly visual displays to reveal previously unseen patterns, relationships, and trends.
But the analytical tools can be complex and highly technical themselves. These tools are for trained technologists and data scientists, not casual users; albeit, software developers are continually striving to simplify the user interface and reduce complexity.
The term "analytics" includes the whole array of tools and applications that parse the data; enable sorting, culling and arrangement; summarize, categorize, and organize data; and provide tools to perform calculations, statistics, modeling, and other mathematical operations. Big Data analytics are based on the data-mining tools that evolved over the last couple of decades in support of data warehousing and data mining. Because of the extent of Big Data (volume, velocity, variety), additional data management functions are required.
Companies that are serious about mining information from Big Data are hiring data scientists to enable the conversation between users (functional managers and decision-makers) and the data and data tools. There can be difficulties in this because data scientists are trained on the technical aspects of data management and analytics but may be inexperienced in business management processes and needs. As a result, the visualizations and analyses presented to users might be according to best practice from an IT standpoint, but might be far less useful from a business perspective.
This is where the importance of having business managers driving the Big Data program is most apparent. Business managers need to think of the conversations they want to have with the data, and be unconstrained by technical or data availability limitations. Data experts and technologists can help in the use case definition by expanding the possibilities, asking "What if" questions (e.g., if you could know this, would it be valuable?).
New capabilities continue to emerge with predictive analytics as a prime focus of recent development efforts. Predictive analytics, as the name implies, are aimed at projecting patterns and trends into the future to provide management with insight into what is likely to occur in the future.
Generally speaking, Big Data and the analytics that we're discussing are primarily outside of the realm of traditional ERP and CRM, although ERP and CRM data are certainly included in analyses. Big Data is mostly external from sensors and devices, partners, customers, social media, and the Internet and is especially useful for monitoring product use and performance, managing costs of operations, creating new sales opportunities in new markets, and uncovering indications for future products and product design improvements, among other things.
Disruptive Models Through IoT
One trend that is having an impact in many facets of manufacturing, including product pricing and profitability, is the Internet of Things (IoT). The IoT generally refers to external data from the Internet, the public, and connected devices "in the wild." The industrial version (IIoT) encompasses smart devices and sensors in the plant and the warehouse. One key aspect of this is machine-to-machine (M2M) connectivity in which sensors feed raw data to smart controllers that can analyze the data and send response (control) signals to equipment and other devices.
M2M provides process controls for quality management and productivity improvement. The raw data feed and/or the resulting information and history of process activities can flow into the Big Data stream to be included with other data sources. This data might be useful in the aforementioned costing/pricing and profitability studies. Some of this detail might also be extremely important for product traceability in relation to meeting regulatory requirements, validating quality and process reliability, and limiting the extent of recalls through detailed recordkeeping.
Whether it's Human Machine Interfaces through evolving mobile technologies and wearable devices, voice or gesture recognition sensors, M2M for machine monitoring, or location-based technologies (GPS), intelligent integration of IoT touch points is changing the way manufacturing companies are creating new business models on the customer-facing end of the business and streamlining operations and increasing throughput internally.
Intelligent integration is an important element of this conversation. Unless the relationships between Big Data & the enterprise & its activities and customers are logically tied together, Big Data will remain "too much of a good thing" & only marginally useful, if at all. To gain the benefits, there must be a clear & concise correlation between Big Data, existing ERP & CRM data, business roles & responsibilities, & the elusive NORA (Non-Obvious Relationship Attribute) components within them. As with any new technology, Big Data analytics is subject to a learning process that may start out as daunting but will quickly grow to be an essential part of day-to-day operations.
A Continuing Challenge
IoT and Big Data offer unprecedented opportunities to better understand products, internal and external performance, market trends, emerging opportunities, customer satisfaction and prospective customer desires, and much more. The biggest challenge in gaining this useful information is that there is just so much data, and a limited capacity to understand it and identify the useful from the useless. Data analysis tools are rapidly evolving to provide more capabilities but this is a race that will probably never reach the finish line. The growth of Big Data is easily outpacing the ability to manage and exploit it. But the growth and maturation of the analytical tools is offering more intelligence and actionable information from Big Data every day.
There are excellent tools available to help exploit Big Data but to be effective, the beautiful and intricate visualizations and analyses must provide intelligence that is to the point and truly useful. The conversation between the human and the commands that direct the analyses is often a Tower of Babel experience where understanding is elusive. That tripping point is not an insurmountable obstacle but it takes attention and patience to get all the players speaking the same language, or at least communicating well enough to guide the analyses in the right direction to provide the information that sparks the next idea or improvement.
Like most other pursuits, exploiting the value that is buried in Big Data is a learning process. The first step is to recognize the opportunities that Big Data brings to the enterprise. Embedding that understanding into the company's DNA opens the door to the conversation.
As an illustration, a snack foods company with data in its DNA wanted to know how consumers really felt about every aspect of certain brands - the size, the taste, the price, the emotional connection, etc. So it combined production data with consumer sentiment data from many sources including social media where it engaged with its most ardent brand fans. The result? Two new and highly successful product launches at the right time and at a price that would sell. Would the ideas have even been introduced without Big Data? We don't know. But we do know that this kind of useful insight is available to any company that can open the conversation by pointing the analytical tools in the right direction for having a conversation with Big Data.
Like most other pursuits, exploiting the value that is buried in Big Data is a learning process.